The increasing in Earth Observation data availability (Landsat & Sentinel systems) and cross-cutting themes reference data (EuroCROPS, WorldCereal, Glance, GBIF), combined with the development of new machine learning approaches (semantic segmentation, auto-encoder, gradient descent tree, autoML) opened several possibilities for producing time-series spatial predictions.
However several challenges are involved in deploying ML models in a production environment considering large amount of data, including: (1) data reading/writing optimization, (2) feature selection, (3) hyper-parameter optimization, (4) time-series reconstruction, and (6) efficient parallelization.
This talk Dr. Leandro Leal Parente will share lessons learned and the computational infrastructure implemented by OpenGeoHub for producing global time-series predictions (grassland, soil carbon, FAPAR, and GPP products) within the context of Open-Earth-Monitor cyberinfrastructure (OEMC) and Global Pasture Watch projects.
Date
11 December 2024, 14:00-15:00 CET
Venue
ITC Building, Hallenweg 8
7522 NH Enschede
LA 2301
or
Online
Speaker
Dr. Leandro Leal Parente
Researcher, OpenGeoHub
Dr. Leandro Leal Parente is a computer scientist with a PhD in Environmental Science working with remote sensing, data science, machine learning, high-performance computing and WebGIS applications. He supports the OpenGeoHub Foundation's projects developing new solutions for geocomputing, optimizing automated workflow to process large-scale Earth Observation data and to model multiple environmental variables through machine learning approaches.
Video
Presentation
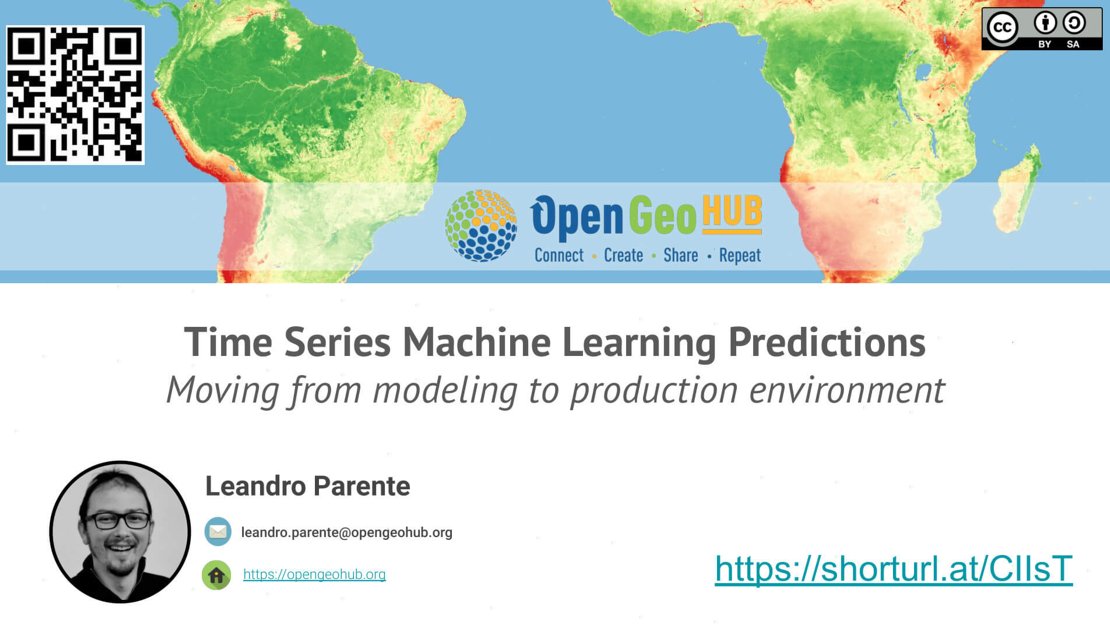
Questions and Answers
Is there any specific strategies for parallelization that can optimize the processing itself? and is there any kind of concern regarding the energy consumptions for processing very large datasets? The first strategy is to split the workflow. Each CPU processes a single pixel. We split processes across the servers, but inside each server, we really try to optimize the workflow. Hence, this is distributed but also parallelized for every server. So most of our infrastructure we manage by ourselves, and we basically have this running Colocation service solution based Ede. We did some research about this service solutions, and they had some green energy certifications.
This answers my question.
Thank you for your feedback
You showed several machine learning techniques, Did you see a significant difference between the models and what's your take from computing platforms or from computing time point of view? and 120,000 hours is for the complete work, which includes all testing and different types of models, right? No, most of the time is spent on predictions. For instance, we have 14 servers, each with 1TB RAM and 96 cores, for Grassland models using Random forest with 60 trees and one model for each class of grassland. We dropped it from 100 to 60 trees which resulted 20-30% percent faster speeds. I would say the random forest is still a bit more computationally and in general produce better results depending on the problem.
This answers my question.
Thank you for your feedback
Do you also consider using CNN deep learning models for your work? We tested some deep learning approach and faced challenges for some variables with scarce data like soil parameters. The main reason for not using deep learning is lack of reference data.
This answers my question.
Thank you for your feedback
Can you provide more information on OpenGeoHub? The summer school starts from 1st to 6th September 2025. We are organizing together with SURF and EO Council. The Summer School will consist of live presentations and demos by leading EO modelers and OSGeo developers, workshops, discussion panels, machine learning competition, and several social events. The summer school will feature an impressive lineup of lecturers and targets PhD, MSc level students and postdocs in the field of geospatial, environmental data, EO data, computer science and computer vision.
This answers my question.
Thank you for your feedback
The workflow and dataset is open but 120000 hours is still a long time. How do you reflect on reproducibilty of platform? The docker image for reproducible environment is publicly available but doing production work would require significant hardware. Hence, people interested in should have infrastructure for their purpose.
This answers my question.
Thank you for your feedback
Is it possible to use and integrate multi-sensor EO data using machine learning with climate and meteorological datasets to assess and predict short- and long-term risks to vulnerable infrastructure? Yeah, it should be possible, but I think that the challenge is really communicating all. One can also have the the models running some small devices and doing some prediction and basically aggregate that in a central system, and there are architectures available.
This answers my question.
Thank you for your feedback